AIS Data Exploration#
First notebook exploring coverage of AIS data. Data was extracted from the UN Global Platform (source data is from Spire / Exact Earth)
Coverage: December 2018 - August 2022
Ports: Tartus, Baniyas, Al Ladhiqiyah. 20 km buffer around each port
import os
from os.path import join
from glob import glob
import pandas as pd
import geopandas as gpd
import folium
from shapely.geometry import Point
import folium.plugins as plugins
import seaborn as sns
from matplotlib import pyplot as plt
pd.options.display.max_columns = None
ais_dir = join(os.path.expanduser("~"), "data", "AIS")
data_dir = join(ais_dir, "Syria")
data_files = glob(data_dir + "/*.csv")
dfs = [pd.read_csv(f, index_col=0) for f in data_files]
df = pd.concat(dfs)
df.head(2)
H3_int_index_6 | message_type | mmsi | dt_insert_utc | longitude | latitude | imo | vessel_name | callsign | vessel_type | vessel_type_code | vessel_type_cargo | vessel_class | length | width | flag_country | flag_code | destination | eta | draught | sog | cog | rot | heading | nav_status | nav_status_code | source | dt_pos_utc | dt_static_utc | vessel_type_main | vessel_type_sub | eeid | source_filename | H3index_0 | H3_int_index_0 | H3_int_index_1 | H3_int_index_2 | H3_int_index_3 | H3_int_index_4 | H3_int_index_5 | H3_int_index_7 | H3_int_index_8 | H3_int_index_9 | H3_int_index_10 | H3_int_index_11 | H3_int_index_12 | H3_int_index_13 | H3_int_index_14 | H3_int_index_15 | polygon_name | hex_resolution | |
---|---|---|---|---|---|---|---|---|---|---|---|---|---|---|---|---|---|---|---|---|---|---|---|---|---|---|---|---|---|---|---|---|---|---|---|---|---|---|---|---|---|---|---|---|---|---|---|---|---|---|---|
90000 | 604285236087357439 | 1 | 355909000.0 | 2020-08-31 23:06:45 | 35.768167 | 35.518667 | 9037276.0 | BEATA | 3FZK3 | Cargo | NaN | NaN | A | 130.0 | 18.0 | Panama | NaN | SY ALD | 8271300.0 | 8.0 | 0.0 | 180.0 | 0.0 | 197.0 | Under Way Using Engine | 0.0 | T-AIS | 2020-08-31 23:06:45 | 2020-08-31 23:06:45 | Oil And Chemical Tanker | Chemical Tanker | NaN | s3a://ungp-ais-data-historical-backup/exact-ea... | 802dfffffffffff | 577269992861466623 | 581769194442326015 | 586271144802254847 | 590774469551718399 | 595278043409285119 | 599781636594204671 | 608788835681173503 | 613292435295961087 | 617796034922020863 | 622299634549325823 | 626803234176679935 | 631306833804357119 | 635810433431727551 | 640314033059097991 | 644817632686468486 | AL LADHIQIYAH | 6 |
90001 | 604285236087357439 | 1 | 355909000.0 | 2020-08-31 22:11:15 | 35.768000 | 35.518833 | 9037276.0 | BEATA | 3FZK3 | Cargo | NaN | NaN | A | 130.0 | 18.0 | Panama | NaN | SY ALD | 8271300.0 | 8.0 | 0.0 | 26.0 | 0.0 | 197.0 | Under Way Using Engine | 0.0 | T-AIS | 2020-08-31 22:11:15 | 2020-08-31 22:11:15 | Oil And Chemical Tanker | Chemical Tanker | NaN | s3a://ungp-ais-data-historical-backup/exact-ea... | 802dfffffffffff | 577269992861466623 | 581769194442326015 | 586271144802254847 | 590774469551718399 | 595278043409285119 | 599781636594204671 | 608788835681173503 | 613292435295961087 | 617796034922020863 | 622299634549325823 | 626803234176671743 | 631306833804040191 | 635810433431410239 | 640314033058780711 | 644817632686151200 | AL LADHIQIYAH | 6 |
df.columns
Index(['H3_int_index_6', 'message_type', 'mmsi', 'dt_insert_utc', 'longitude',
'latitude', 'imo', 'vessel_name', 'callsign', 'vessel_type',
'vessel_type_code', 'vessel_type_cargo', 'vessel_class', 'length',
'width', 'flag_country', 'flag_code', 'destination', 'eta', 'draught',
'sog', 'cog', 'rot', 'heading', 'nav_status', 'nav_status_code',
'source', 'dt_pos_utc', 'dt_static_utc', 'vessel_type_main',
'vessel_type_sub', 'eeid', 'source_filename', 'H3index_0',
'H3_int_index_0', 'H3_int_index_1', 'H3_int_index_2', 'H3_int_index_3',
'H3_int_index_4', 'H3_int_index_5', 'H3_int_index_7', 'H3_int_index_8',
'H3_int_index_9', 'H3_int_index_10', 'H3_int_index_11',
'H3_int_index_12', 'H3_int_index_13', 'H3_int_index_14',
'H3_int_index_15', 'polygon_name', 'hex_resolution'],
dtype='object')
Check unique vessel ids
df.loc[df.loc[:, "mmsi"].isna(), "mmsi"] = -1
df.loc[:, "mmsi"] = df.loc[:, "mmsi"].astype("int")
len(df), len(df.mmsi.unique())
(98662, 498)
Ports from WPI
wpi = pd.read_csv(join(ais_dir, "wpi", "UpdatedPub150.csv"))
wpi = wpi.loc[wpi["Country Code"] == "Syria"].copy()
geoms = [Point(xy) for xy in zip(wpi.Longitude, wpi.Latitude)]
wpi_gdf = gpd.GeoDataFrame(wpi, crs="EPSG:4326", geometry=geoms)
wpi_gdf = wpi_gdf.to_crs("EPSG:32636")
wpi_gdf.loc[:, "geometry"] = wpi_gdf.buffer(20000) # 10000
wpi_gdf = wpi_gdf.to_crs("EPSG:4326")
wpi_gdf
World Port Index Number | Region Name | Main Port Name | Alternate Port Name | UN/LOCODE | Country Code | World Water Body | IHO S-130 Sea Area | Sailing Direction or Publication | Standard Nautical Chart | ... | Supplies - Diesel Oil | Supplies - Aviation Fuel | Supplies - Deck | Supplies - Engine | Repairs | Dry Dock | Railway | Latitude | Longitude | geometry | |
---|---|---|---|---|---|---|---|---|---|---|---|---|---|---|---|---|---|---|---|---|---|
422 | 45003 | Syria -- 45000 | Tartus | SY TTS | Syria | Mediterranean Sea; North Atlantic Ocean | Sailing Directions Pub. 132 (Enroute) - Easter... | 56048 | ... | Unknown | Unknown | Unknown | Unknown | Limited | Medium | Medium | 34.900000 | 35.866667 | POLYGON ((36.08527 34.89464, 36.08356 34.87702... | ||
861 | 45005 | Syria -- 45000 | Baniyas | SY BAN | Syria | Mediterranean Sea; North Atlantic Ocean | Sailing Directions Pub. 132 (Enroute) - Easter... | 56047 | ... | Yes | Unknown | Unknown | Unknown | Limited | Unknown | Unknown | 35.183333 | 35.950000 | POLYGON ((36.16934 35.17779, 36.16760 35.16016... | ||
1367 | 45010 | Syria -- 45000 | Al Ladhiqiyah | Latakia | SY ALD | Syria | Mediterranean Sea; North Atlantic Ocean | Sailing Directions Pub. 132 (Enroute) - Easter... | 56048 | ... | Yes | Unknown | Yes | Yes | Limited | Large | Medium | 35.533333 | 35.766667 | POLYGON ((35.98700 35.52807, 35.98528 35.51045... |
3 rows × 108 columns
wpi_gdf.loc[:, "color"] = ["blue", "orange", "green"]
def style_function(feature):
idx = int(feature["id"])
color = wpi_gdf.loc[idx, "color"]
return {"fillOpacity": 0.2, "weight": 0, "fillColor": color}
gdf_col = wpi_gdf.geometry.envelope.__geo_interface__
# df_sample = df.head(1000).copy()
df_sample = df.copy()
df_sample.loc[:, "bool"] = 1
[lat, lon] = buffer_df[["Latitude", "Longitude"]].iloc[0].tolist()
m = folium.Map(
# location = [-8.102782 ,156.833900 ],
location=[lat, lon],
tiles="cartodbpositron",
zoom_start=8,
)
a = plugins.HeatMap(
df_sample[["latitude", "longitude"]].values, radius=2, blur=2, name="HeatMap"
).add_to(m)
a = folium.GeoJson(gdf_col, name="BBox", style_function=style_function).add_to(m)
folium.LayerControl().add_to(m)
m
Make this Notebook Trusted to load map: File -> Trust Notebook
df_mmsi = df.copy()
df_mmsi.drop_duplicates("mmsi", inplace=True)
len(df_mmsi)
498
list(df_mmsi.columns)
['H3_int_index_6',
'message_type',
'mmsi',
'dt_insert_utc',
'longitude',
'latitude',
'imo',
'vessel_name',
'callsign',
'vessel_type',
'vessel_type_code',
'vessel_type_cargo',
'vessel_class',
'length',
'width',
'flag_country',
'flag_code',
'destination',
'eta',
'draught',
'sog',
'cog',
'rot',
'heading',
'nav_status',
'nav_status_code',
'source',
'dt_pos_utc',
'dt_static_utc',
'vessel_type_main',
'vessel_type_sub',
'eeid',
'source_filename',
'H3index_0',
'H3_int_index_0',
'H3_int_index_1',
'H3_int_index_2',
'H3_int_index_3',
'H3_int_index_4',
'H3_int_index_5',
'H3_int_index_7',
'H3_int_index_8',
'H3_int_index_9',
'H3_int_index_10',
'H3_int_index_11',
'H3_int_index_12',
'H3_int_index_13',
'H3_int_index_14',
'H3_int_index_15',
'polygon_name',
'hex_resolution']
df_mmsi.vessel_type.value_counts()
Cargo 373
Tanker 47
Tug 18
Unknown 13
UNAVAILABLE 13
Fishing 8
Other 5
Passenger 5
Towing 4
SAR 2
WIG 2
Port Tender 2
Reserved 1
Dredging 1
Diving 1
Law Enforcement 1
Pleasure Craft 1
Pilot 1
Name: vessel_type, dtype: int64
df_mmsi.vessel_type_main.value_counts()
General Cargo Ship 107
Bulk Carrier 67
Container Ship 49
Oil And Chemical Tanker 26
Ro Ro Cargo Ship 16
Specialized Cargo Ship 11
Tug 8
Fishing Vessel 4
Service Ship 4
Offshore Vessel 3
Other Tanker 3
Gas Tanker 3
Other 1
Pleasure Craft 1
Name: vessel_type_main, dtype: int64
df_mmsi.flag_country.value_counts()
Togo 61
Panama 48
Comoros 35
Turkey 33
Russian Federation 31
Liberia 25
Malta 24
Palau 16
Marshall Islands 16
Belize 15
Tanzania 15
Iran 12
Sierra Leone 11
Antigua and Barbuda 11
Japan 11
Egypt 10
Moldova 10
Lebanon 8
Italy 7
Cyprus 7
Norway 7
Syria 7
Singapore 6
Saint Kitts and Nevis 5
Cameroon 5
South Korea 5
China 5
USA 4
Bahamas 4
Mozambique 3
Netherlands 3
Guyana 3
Saint Vincent and the Grenadines 2
Gibraltar 2
Greece 2
Hong Kong 2
Cayman Islands 2
Denmark 2
Cook Islands 2
Vanuatu 2
Tuvalu 2
Germany 1
Taiwan 1
Barbados 1
Ireland 1
Mongolia 1
Equatorial Guinea 1
Poland 1
Zambia 1
Luxembourg 1
Brazil 1
Honduras 1
Spain 1
Madeira 1
Israel 1
Name: flag_country, dtype: int64
df_mmsi.loc[df_mmsi.flag_country == "Togo", "mmsi"]
90067 671350000
90071 671700000
90088 671032000
90216 671158100
90270 671259100
...
61456 671211100
71028 671218100
73071 671265000
76527 671662000
88155 671456000
Name: mmsi, Length: 61, dtype: int64
df.loc[
df.mmsi == 671700000,
[
"message_type",
"mmsi",
"dt_insert_utc",
"longitude",
"latitude",
"imo",
"vessel_name",
"callsign",
"vessel_type",
"vessel_type_code",
"vessel_type_cargo",
"vessel_class",
"length",
"width",
"flag_country",
"flag_code",
"destination",
"eta",
"draught",
"sog",
"cog",
"rot",
"heading",
"nav_status",
"nav_status_code",
"source",
"dt_pos_utc",
"dt_static_utc",
"vessel_type_main",
"vessel_type_sub",
"eeid",
],
].head()
message_type | mmsi | dt_insert_utc | longitude | latitude | imo | vessel_name | callsign | vessel_type | vessel_type_code | vessel_type_cargo | vessel_class | length | width | flag_country | flag_code | destination | eta | draught | sog | cog | rot | heading | nav_status | nav_status_code | source | dt_pos_utc | dt_static_utc | vessel_type_main | vessel_type_sub | eeid | |
---|---|---|---|---|---|---|---|---|---|---|---|---|---|---|---|---|---|---|---|---|---|---|---|---|---|---|---|---|---|---|---|
90071 | 27 | 671700000 | 2021-11-15 18:28:13 | 35.87 | 34.901667 | 7405089.0 | PRINCESS HIYAM | 5VDW8 | Unknown | 100.0 | NaN | A | 79.0 | 13.0 | Togo | 671.0 | NaN | 2460.0 | 0.0 | 0.0 | 248.0 | 0.0 | 0.0 | Moored | 5.0 | S-AIS | 2021-11-15 18:27:55 | 2021-10-12 18:33:34 | Specialized Cargo Ship | Livestock Carrier | 4.686739e+18 |
90072 | 27 | 671700000 | 2021-11-15 06:10:16 | 35.87 | 34.901667 | 7405089.0 | PRINCESS HIYAM | 5VDW8 | Unknown | 100.0 | NaN | A | 79.0 | 13.0 | Togo | 671.0 | NaN | 2460.0 | 0.0 | 0.0 | 289.0 | 0.0 | 0.0 | Moored | 5.0 | S-AIS | 2021-11-15 06:09:57 | 2021-10-12 18:33:34 | Specialized Cargo Ship | Livestock Carrier | 4.686739e+18 |
90073 | 27 | 671700000 | 2021-11-15 04:16:17 | 35.87 | 34.901667 | 7405089.0 | PRINCESS HIYAM | 5VDW8 | Unknown | 100.0 | NaN | A | 79.0 | 13.0 | Togo | 671.0 | NaN | 2460.0 | 0.0 | 0.0 | 292.0 | 0.0 | 0.0 | Moored | 5.0 | S-AIS | 2021-11-15 04:15:54 | 2021-10-12 18:33:34 | Specialized Cargo Ship | Livestock Carrier | 4.686739e+18 |
90074 | 27 | 671700000 | 2021-11-15 04:58:13 | 35.87 | 34.901667 | 7405089.0 | PRINCESS HIYAM | 5VDW8 | Unknown | 100.0 | NaN | A | 79.0 | 13.0 | Togo | 671.0 | NaN | 2460.0 | 0.0 | 0.0 | 261.0 | 0.0 | 0.0 | Moored | 5.0 | S-AIS | 2021-11-15 04:57:56 | 2021-10-12 18:33:34 | Specialized Cargo Ship | Livestock Carrier | 4.686739e+18 |
90477 | 27 | 671700000 | 2021-11-16 05:13:11 | 35.87 | 34.901667 | 7405089.0 | PRINCESS HIYAM | 5VDW8 | Unknown | 100.0 | NaN | A | 79.0 | 13.0 | Togo | 671.0 | NaN | 2460.0 | 0.0 | 0.0 | 281.0 | 0.0 | 0.0 | Moored | 5.0 | S-AIS | 2021-11-16 05:12:58 | 2021-10-12 18:33:34 | Specialized Cargo Ship | Livestock Carrier | 4.686739e+18 |
df.loc[:, "dt_pos_utc"] = pd.to_datetime(df.dt_insert_utc)
df.loc[:, "day"] = df.loc[:, "dt_pos_utc"].dt.strftime("%Y-%m-%d")
df.loc[:, "month"] = df.loc[:, "dt_pos_utc"].dt.strftime("%Y-%m")
df[["mmsi", "day", "month"]].head()
mmsi | day | month | |
---|---|---|---|
90000 | 355909000 | 2020-08-31 | 2020-08 |
90001 | 355909000 | 2020-08-31 | 2020-08 |
90002 | 355909000 | 2020-08-31 | 2020-08 |
90003 | 355909000 | 2020-08-31 | 2020-08 |
90004 | 355909000 | 2020-08-31 | 2020-08 |
df_raw = df.copy()
df = df_raw.copy()
df_mmsi.vessel_type.unique()
array(['Cargo', 'Tug', 'Unknown', 'Tanker', 'Towing', 'UNAVAILABLE',
'SAR', 'Other', 'Reserved', 'Passenger', 'Fishing', 'Dredging',
'WIG', 'Diving', 'Law Enforcement', 'Port Tender',
'Pleasure Craft', 'Pilot'], dtype=object)
df_mmsi.vessel_type_main.unique()
array(['Oil And Chemical Tanker', 'Container Ship', nan,
'General Cargo Ship', 'Bulk Carrier', 'Specialized Cargo Ship',
'Ro Ro Cargo Ship', 'Offshore Vessel', 'Other', 'Tug',
'Other Tanker', 'Fishing Vessel', 'Service Ship', 'Gas Tanker',
'Pleasure Craft'], dtype=object)
accepted = ["Cargo"]
# accepted = ['Oil And Chemical Tanker', 'Container Ship',
# 'General Cargo Ship', 'Bulk Carrier', 'Specialized Cargo Ship',
# 'Ro Ro Cargo Ship', 'Other Tanker', 'Gas Tanker']
df = df.loc[df.vessel_type.isin(accepted)].copy()
# df = df.loc[df.vessel_type_main.isin(accepted)].copy()
df.vessel_type_main.unique()
array(['Oil And Chemical Tanker', 'Container Ship', nan,
'General Cargo Ship', 'Bulk Carrier', 'Ro Ro Cargo Ship',
'Specialized Cargo Ship', 'Offshore Vessel', 'Other',
'Service Ship', 'Fishing Vessel', 'Tug'], dtype=object)
df_raw.nav_status.value_counts()
Moored 43015
Under Way Using Engine 27537
At Anchor 22841
Not Under Command 2823
Underway Sailing 1653
Restricted Manoeuvrability 565
Not Defined 160
Engaged In Fishing 41
Unknown 27
Name: nav_status, dtype: int64
df.sort_values("day").head()
H3_int_index_6 | message_type | mmsi | dt_insert_utc | longitude | latitude | imo | vessel_name | callsign | vessel_type | vessel_type_code | vessel_type_cargo | vessel_class | length | width | flag_country | flag_code | destination | eta | draught | sog | cog | rot | heading | nav_status | nav_status_code | source | dt_pos_utc | dt_static_utc | vessel_type_main | vessel_type_sub | eeid | source_filename | H3index_0 | H3_int_index_0 | H3_int_index_1 | H3_int_index_2 | H3_int_index_3 | H3_int_index_4 | H3_int_index_5 | H3_int_index_7 | H3_int_index_8 | H3_int_index_9 | H3_int_index_10 | H3_int_index_11 | H3_int_index_12 | H3_int_index_13 | H3_int_index_14 | H3_int_index_15 | polygon_name | hex_resolution | day | month | |
---|---|---|---|---|---|---|---|---|---|---|---|---|---|---|---|---|---|---|---|---|---|---|---|---|---|---|---|---|---|---|---|---|---|---|---|---|---|---|---|---|---|---|---|---|---|---|---|---|---|---|---|---|---|
0 | 604285235818921983 | 1 | 312945000 | 2018-12-02 19:31:21 | 35.759750 | 35.526542 | 9197935.0 | TRUEBORN | V3XY4 | Cargo | NaN | NaN | A | 171.0 | 27.0 | Belize | NaN | ODESA | 12271800.0 | 6.0 | 0.1 | 241.3 | 0.0 | 78.0 | Under Way Using Engine | 0.0 | S-AIS | 2018-12-02 19:31:21 | 2018-12-02 19:31:21 | Bulk Carrier | NaN | NaN | s3a://ungp-ais-data-historical-backup/exact-ea... | 802dfffffffffff | 577269992861466623 | 581769194442326015 | 586271144802254847 | 590774469551718399 | 595278043409285119 | 599781636594204671 | 608788835681173503 | 613292435304349695 | 617796034930147327 | 622299634557386751 | 626803234184744959 | 631306833812112895 | 635810433439485183 | 640314033066853679 | 644817632694224173 | AL LADHIQIYAH | 6 | 2018-12-02 | 2018-12 |
1 | 604285235818921983 | 1 | 312945000 | 2018-12-03 07:09:27 | 35.759750 | 35.526500 | 9197935.0 | TRUEBORN | V3XY4 | Cargo | NaN | NaN | A | 171.0 | 27.0 | Belize | NaN | POR SAID | 12271800.0 | 6.0 | 0.0 | 309.0 | 0.0 | 78.0 | Under Way Using Engine | 0.0 | S-AIS | 2018-12-03 07:09:27 | 2018-12-03 07:09:27 | Bulk Carrier | NaN | NaN | s3a://ungp-ais-data-historical-backup/exact-ea... | 802dfffffffffff | 577269992861466623 | 581769194442326015 | 586271144802254847 | 590774469551718399 | 595278043409285119 | 599781636594204671 | 608788835681173503 | 613292435304349695 | 617796034930147327 | 622299634557386751 | 626803234184744959 | 631306833812112895 | 635810433439483199 | 640314033066853639 | 644817632694224134 | AL LADHIQIYAH | 6 | 2018-12-03 | 2018-12 |
3 | 604285236087357439 | 1 | 312945000 | 2018-12-05 10:00:35 | 35.767700 | 35.517750 | 9197935.0 | TRUEBORN | V3XY4 | Cargo | NaN | NaN | A | 171.0 | 27.0 | Belize | NaN | POR SAID | 12271800.0 | 6.0 | 0.0 | 141.0 | 0.0 | 198.0 | Under Way Using Engine | 0.0 | S-AIS | 2018-12-05 10:00:35 | 2018-12-05 10:00:35 | Bulk Carrier | NaN | NaN | s3a://ungp-ais-data-historical-backup/exact-ea... | 802dfffffffffff | 577269992861466623 | 581769194442326015 | 586271144802254847 | 590774469551718399 | 595278043409285119 | 599781636594204671 | 608788835681173503 | 613292435295961087 | 617796034922020863 | 622299634549161983 | 626803234176507903 | 631306833803876863 | 635810433431246911 | 640314033058617391 | 644817632685987886 | AL LADHIQIYAH | 6 | 2018-12-05 | 2018-12 |
2 | 604285235818921983 | 3 | 636092669 | 2018-12-05 18:37:52 | 35.768033 | 35.526600 | 9430870.0 | HANSA NEUBURG | D5KB8 | Cargo | NaN | NaN | A | 175.0 | 27.0 | Liberia | NaN | PIRAEUS | 12281400.0 | 7.9 | 0.0 | 101.0 | 0.0 | 228.0 | Moored | 5.0 | S-AIS | 2018-12-05 18:37:52 | 2018-12-05 18:37:52 | Container Ship | NaN | NaN | s3a://ungp-ais-data-historical-backup/exact-ea... | 802dfffffffffff | 577269992861466623 | 581769194442326015 | 586271144802254847 | 590774469551718399 | 595278043409285119 | 599781636594204671 | 608788835362406399 | 613292434987679743 | 617796034613739519 | 622299634240913407 | 626803233868279807 | 631306833495649279 | 635810433123019391 | 640314032750389855 | 644817632377760348 | AL LADHIQIYAH | 6 | 2018-12-05 | 2018-12 |
4 | 604285235818921983 | 1 | 271044633 | 2018-12-06 23:16:32 | 35.735950 | 35.536378 | 9106479.0 | MED CORLU | TCA4362 | Cargo | NaN | NaN | A | 0.0 | 0.0 | Turkey | NaN | LBBEY | 12302200.0 | 6.1 | 3.9 | 74.6 | 0.0 | 92.0 | Under Way Using Engine | 0.0 | S-AIS | 2018-12-06 23:16:32 | 2018-12-06 23:16:32 | Container Ship | NaN | NaN | s3a://ungp-ais-data-historical-backup/exact-ea... | 802dfffffffffff | 577269992861466623 | 581769194442326015 | 586271144802254847 | 590774469551718399 | 595278043409285119 | 599781636594204671 | 608788835429515263 | 613292435054788607 | 617796034681634815 | 622299634308775935 | 626803233936117759 | 631306833563487231 | 635810433190857535 | 640314032818227999 | 644817632445598494 | AL LADHIQIYAH | 6 | 2018-12-06 | 2018-12 |
# df_port = df[['mmsi', 'day', 'polygon_name']].groupby(['polygon_name', 'day']).nunique()
df_port = (
df[["mmsi", "month", "polygon_name"]].groupby(["polygon_name", "month"]).nunique()
)
df_port.reset_index(inplace=True)
# df_port.loc[:, 'date'] = pd.to_datetime(df_port.day)
df_port.loc[:, "date"] = pd.to_datetime(df_port.month)
df_port = df_port.loc[df_port.polygon_name != "BANIYAS"]
# sns.set(font_scale = 1.5) # sns.set_style("white")
sns.set_theme(style="whitegrid", font_scale=1.5)
fig, ax = plt.subplots(figsize=(11, 6))
ax = sns.lineplot(data=df_port, x="date", y="mmsi", hue="polygon_name", palette="tab10")
ax.set(xlabel="", ylabel="Unique MMSI", title="Number of unique vessels per month")
plt.legend(title="") # loc='upper right', labels=['Raw Data', 'Interpolated']
# plt.savefig("../docs/images/Output_Mining_Gas.png", facecolor='white', dpi=300)
<matplotlib.legend.Legend at 0x7f5614034f10>
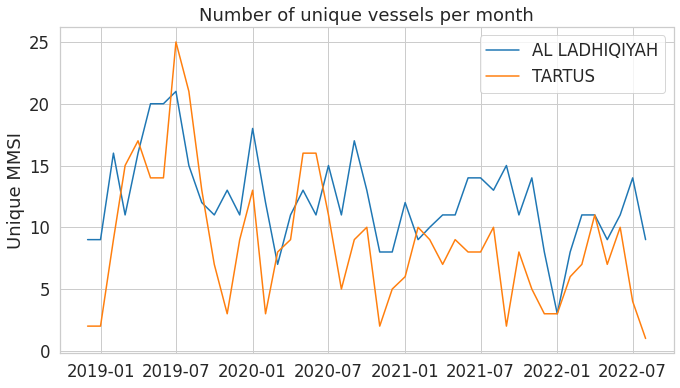
fig, ax = plt.subplots(figsize=(11, 6))
ax = sns.lineplot(data=df_port, x="date", y="mmsi", hue="polygon_name", palette="tab10")
ax.set(xlabel="", ylabel="Unique MMSI", title="Number of unique vessels per month")
plt.legend(title="") # loc='upper right', labels=['Raw Data', 'Interpolated']
# plt.savefig("../docs/images/Output_Mining_Gas.png", facecolor='white', dpi=300)
<matplotlib.legend.Legend at 0x7f5614d77280>
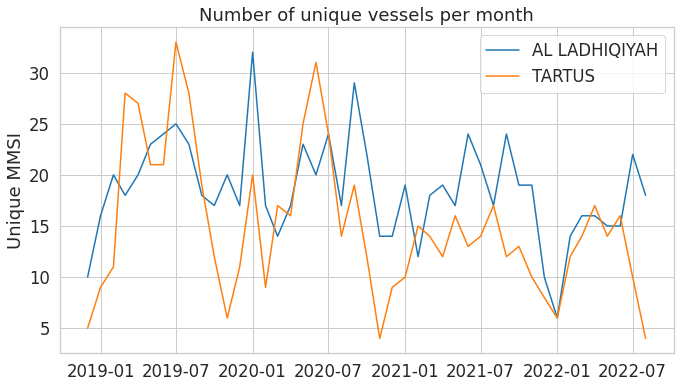